Participation: by invitation ONLY
Application Deadline: 27 May 2024 (Monday)
Programme Rundown
9:00 am – 9:10 am Opening Remarks
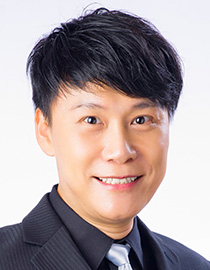
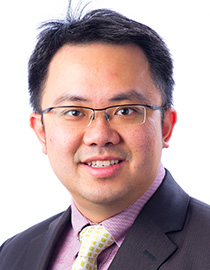
9:10 am – 10:00 am

10:00 am – 10:45 am
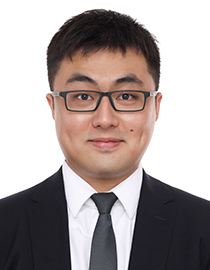
10:45 am – 11:00 am Break
11:00 am – 11:50 am

11:50 am – 12:30 pm
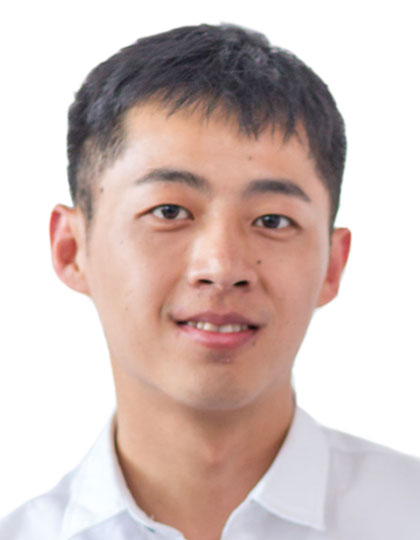
Prof Menglong LI
View Abstract
12:30 pm – 2:30 pm Lunch
2:30 pm – 3:20 pm
Prof Ming HU
View Abstract
3:20 pm – 3:50 pm
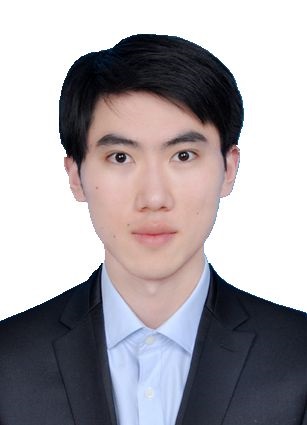
Tianqi SONG (CityU)
View Abstract
3:50 pm – 4:05 pm Break
4:05 pm – 4:35 pm
Qiaochu FU
View Abstract
4:35 pm – 5:05 pm
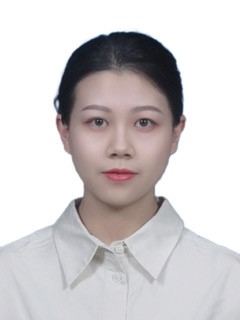
Dr. Xiaoxiao LI
View Abstract
5:05 pm – 5:30 pm Interactive Session with Speakers
Abstract
Prof Peng SUN
Optimal Conditional Drug Approval
New prescription drugs require regulatory approval before drug makers can sell them. In some countries, regulators may conditionally approve a drug, which allows sales to begin before the developer has proven the drug's efficacy. After further testing, the regulator may either grant final approval or reject the drug. We show that conditional approval not only speeds access to drugs but also encourages the development of drugs that would not have been pursued otherwise. Using mechanism design principles, we show that regulators should conditionally approve a drug even if it is ex-ante less likely to prove efficacious, under certain conditions. The regulator should approve the drug to encourage investment, especially when only the firm knows the testing cost. However, drugs that are less likely to prove efficacious should only be conditionally approved for a portion of the patient population if that is enough to motivate testing. Additionally, the impact of conditional approval is greater when the firm's revenue increases over time. Finally, a regulator should sometimes commit that in the future it will grant final approval for a drug that narrowly misses the efficacy threshold in return for the firm testing an otherwise unprofitable drug.
Prof Tim HUH
Performance of the offer-everything policy
We study a sequential assortment optimization problem over a finite selling horizon with an exogenously fixed initial inventory for multiple products. The manager offers an assortment in each period. The assortment cannot depend on arriving customer types because the manager does not see the customer type before making the assortment decision and thus cannot treat customer types differently. Focusing on an online setting where all products have the same price, we show a competitive ratio cannot be higher than 1/2 against an adversary. This adversary knows the sequence of arrivals and exactly which product each customer purchases depending on the assortment offered. We show that a commonly-used policy that offers all available products in each period achieves a competitive ratio of 1/2, matching the upper bound. In fact, we prove a stronger result. For each problem instance, this policy achieves the best worst-case performance over all possible customer arrival sequences. Finally, we discuss extensions to non-identical prices and randomized policies.
Joint work with Joseph Paat and Maurice Queyranne.
Prof Ming HU
Randomization in Product, Fulfilment, and Pricing Strategies as a Profit Lever
First, we study blind boxes as a novel selling mechanism in which buyers purchase sealed packages containing unknown items, with the chance of uncovering rare or special items. We show how such product randomization introduced by the blind box can improve the seller’s profitability over traditional separate selling. Second, we study how an e-commerce platform should assign sequentially arriving customers to sellers who compete to sell identical products on the platform. The allocation rule may be random and dependent on the sellers’ inventory levels. We show how such demand fulfillment randomization can incentivize sellers to hold more inventory and improve the platform’s profitability and customer welfare. Third, we study randomized promotions in which the firm randomly offers discounts over time to sequentially arriving customers with heterogeneous valuations and patience levels. We show how price randomization can improve the firm’s profitability beyond deterministic pricing policies.
Prof Hanwei LI
Data-Driven Real-time Coupon Allocation in Online Platform
In this study, we partner with Meituan, a leading shopping platform, to develop a real-time, end-to-end coupon allocation system that aims to maximize customer conversion rate (CVR) while adhering to marketing budgets, addressing challenges of uncertain traffic from a diverse customer base and the need for real-time decision-making. By leveraging comprehensive customer and product features, we estimate CVR under various coupon values and employ isotonic regression to ensure non-increasing predicted CVRs with respect to price. Using calibrated CVR predictions as input, we propose a Lagrangian Dual-based algorithm that efficiently determines optimal coupon values for individual customers upon arrival in O(N) computation time. We assess the effectiveness of our coupon allocation algorithm through a field experiment and historical data. So far, our framework has distributed coupons to over 100 million users in more than 110 major cities in China and increased revenue by 0.7%, which translates to an additional 8 million RMB annual profit for the platform compared to the traditional, offline IP approach.
Prof Menglong LI
Asymptotic Optimality of Open-Loop Policies in Lost-Sales Inventory Models with Stochastic Lead Times
Inventory models with lost sales and large lead times are notoriously difficult to manage due to the curse of dimensionality. In this talk, we consider the lost-sales inventory model in which the lead time is not only large but also random. Under the assumption that the placed orders cannot cross in time, we establish the asymptotic optimality of constant-order policies as the lead time increases for the model with divisible products.
For the model with indivisible products, we propose an open-loop bracket policy, which alternates deterministically between two consecutive integer order quantities. By employing the concept of multimodularity, we prove that the bracket policy is asymptotically optimal.
Our results on divisible products also hold for the models with order crossover and random supply functions. As our main methodological contributions, we establish the convergence of the average cost incurred by the optimal open-loop policy in a finite-period problem, which serves as a lower bound of the optimal long-run average cost, to the long-run average cost generated by our proposed open-loop policies.
Finally, we provide a numerical study to derive further insights, and find out that the proposed open-loop policies perform well even for short lead times when the ratio between the lost-sales penalty cost and holding cost is moderate.
Tianqi SONG, Prof Biying SHOU, Prof Pengfei GUO
Bayesian Insights into the Government-Retailer Information Disclosure during Panic Buying
When facing a potential supply disruption risk, consumers receive information from various sources, such as retailers and governments. It is crucial for retailers and governments to understand how strategic disclosure of information influences consumer behavior. In this paper, we analyze the optimal information designs of retailers and governments during panic buying through a Bayesian persuasion framework. By modeling both simultaneous and sequential information disclosure processes, we derive their optimal decisions for different scenarios and the impacts on consumer behavior and the supply chain. Our findings reveal that simultaneous disclosure typically leads to full transparency, while sequential disclosure, especially when the government sends signals first, allows for partial disclosure, resulting in better outcomes for both the government and retailers. This study highlights the importance of strategic information management in crisis scenarios and provides key insights into optimizing information dissemination to mitigate economic and social impacts.
Qiaochu FU
Joint Assortment Planning and Inventory Control under General Choice Models
We give an approximation framework for the joint assortment planning and inventory management problem and derive a constant-factor guarantee for a broad class of choice models, including the Markov chain choice model. Our algorithm starts from a fluid approximation to identify a profitable assortment, and use rounding and greedy heuristic method to decide the inventory level of the products restricted to the profitable assortment. Our approach outperforms the existing methods both theoretically and experimentally.
Dr Xiaoxiao LI
Cyclic Pricing for Live-Streaming Selling: the Role of Herding Behaviors
We consider continuous-time dynamic pricing in the presence of waiting customers for live-streaming selling with herding behaviors. Customers arrive at a constant rate over time with heterogeneous valuations. Customers purchase if the current price is less than their corresponding valuations. The remaining customers may leave or wait for a future live-streaming sale. The waiting customers exhibit a variety of herding behaviors when making waiting decisions, where these herding behaviors are characterized by the population-contingent leaving probability. Leveraging the waiting customers' behaviors, the seller makes the pricing decision with a finite menu of prices each time and has the option to offer a lowest price to attract all waiting customers through a live-streaming sale. We build a continuous-time model by exploiting impulse control. We show that the optimal dynamic pricing policy can be either a pump and dump or generalized pump and dump cyclic pricing strategy, determined by the effective marginal population-contingent leaving rate. Moreover, the seller has no incentive to offer a regular price which is smaller than the myopic optimal price. If customers exhibit reverse-, non-, or weak-herding behavior for waiting, a pump and dump cyclic pricing strategy is optimal. On the other hand, if they have the strong-herding behavior, a generalized pump and dump cyclic pricing strategy becomes optimal. We provide analytical solutions for the cycle length and the optimal pricing path. Our findings provide guidelines on how to carry out dynamic pricing for live-stream selling. Numerically, we show that ignoring cyclic pricing can lead to a significant revenue loss.
Registration by invitation only!
For details, please contact Prof Frank Chen (youhchen@cityu.edu.hk) or Miss Frances Au (Frances.Au@cityu.edu.hk)